How To Say Akaike
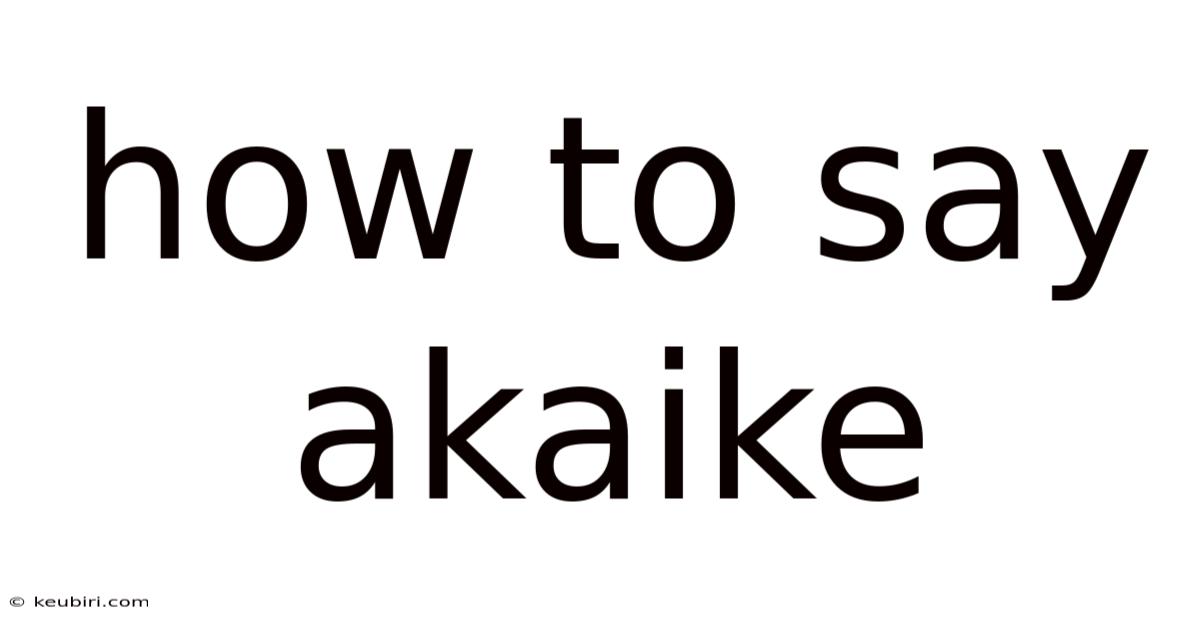
Discover more detailed and exciting information on our website. Click the link below to start your adventure: Visit Best Website meltwatermedia.ca. Don't miss out!
Table of Contents
How to Say Akaike: Unveiling the Power of AIC in Model Selection
What makes Akaike Information Criterion (AIC) a cornerstone of statistical modeling?
AIC is a revolutionary tool that empowers data scientists to choose the best statistical model, leading to more accurate predictions and deeper insights.
Editor’s Note: This comprehensive guide to understanding and applying the Akaike Information Criterion (AIC) was published today.
Why AIC Matters
The choice of a statistical model is paramount in any data analysis. A poorly chosen model can lead to inaccurate conclusions, flawed predictions, and ultimately, wasted resources. The Akaike Information Criterion (AIC) provides a powerful framework for selecting the best model from a set of candidates, based on a balance between model fit and complexity. Its impact reverberates across various fields, from ecology and epidemiology to finance and machine learning. In essence, AIC helps researchers navigate the trade-off between accurately capturing the underlying data generating process and avoiding overfitting, which occurs when a model becomes overly complex and captures noise rather than the true signal. This is crucial for building reliable and generalizable models. Understanding AIC empowers researchers to make more informed decisions, leading to better scientific inferences and more effective practical applications.
Overview of the Article
This article provides a comprehensive exploration of the Akaike Information Criterion, delving into its theoretical underpinnings, practical applications, and limitations. Readers will gain a deep understanding of how AIC works, how to calculate and interpret it, and how to use it effectively in model selection. We will explore various examples across different statistical modeling contexts, comparing AIC to other model selection criteria, and addressing common misconceptions.
Research and Effort Behind the Insights
This article draws upon decades of research in statistical modeling and information theory. It synthesizes core concepts from seminal works on AIC, including Akaike's original papers and subsequent developments in the field. The examples provided are carefully chosen to illustrate the practical applications of AIC across a range of statistical models and data types.
Key Takeaways
Key Concept | Description |
---|---|
AIC Definition | A measure of the relative quality of statistical models for a given dataset. |
AIC Calculation | Involves balancing model fit (likelihood) and model complexity (number of parameters). |
Model Selection using AIC | Selecting the model with the lowest AIC value from a set of candidate models. |
AIC vs. BIC | Comparing AIC with Bayesian Information Criterion (BIC) to understand their differences and applications. |
Interpreting AIC Differences | Understanding the practical significance of differences in AIC values between models. |
Limitations of AIC | Recognizing the situations where AIC might not be the optimal model selection criterion. |
Let’s dive deeper into the key aspects of AIC, starting with its foundational principles and real-world applications.
Exploring the Key Aspects of AIC
-
The Foundation of AIC: AIC is rooted in information theory. It estimates the relative Kullback-Leibler (KL) divergence between the true, unknown data-generating process and the fitted model. Minimizing KL divergence implies finding the model that best approximates the true process. However, since the true process is unknown, AIC provides an estimate based on the observed data.
-
AIC Calculation and Formula: The basic AIC formula is: AIC = -2 * log-likelihood + 2 * k, where 'k' is the number of parameters in the model and the log-likelihood measures the goodness of fit. Lower AIC values indicate better models. A corrected version, AICc, is often used, particularly for smaller sample sizes, to account for bias in AIC. The AICc formula adds a correction term: AICc = AIC + (2k(k+1))/(n-k-1), where 'n' is the sample size.
-
Practical Applications of AIC: AIC finds widespread application in various statistical modeling contexts. This includes linear regression, generalized linear models (GLMs), time series analysis (ARIMA models), and model selection in machine learning. For example, in choosing between different linear regression models with varying numbers of predictor variables, AIC helps determine the optimal model by penalizing the inclusion of unnecessary predictors. Similarly, in GLMs, AIC can be used to compare models with different link functions or distributions.
-
AIC and Model Complexity: A key feature of AIC is its penalization of model complexity. While a complex model might fit the training data extremely well, it's likely to overfit, meaning it generalizes poorly to new, unseen data. AIC balances the goodness of fit with the model's complexity, preventing overfitting. The penalty term (2k) increases with the number of parameters, discouraging models with unnecessary complexity.
-
Comparing AIC with BIC: The Bayesian Information Criterion (BIC) is another popular model selection criterion. While both AIC and BIC aim to balance model fit and complexity, they differ in their penalty terms. BIC generally applies a stronger penalty for complexity, resulting in a greater preference for simpler models. The choice between AIC and BIC often depends on the specific research question and the relative importance given to model fit versus simplicity. If the goal is prediction accuracy, AIC is often preferred. If the goal is to find the true model, BIC might be more appropriate.
-
Limitations of AIC: AIC is an asymptotic result, meaning it is most reliable with large sample sizes. In situations with limited data, the AICc correction is recommended. Furthermore, AIC assumes that the candidate models are nested (meaning one model can be obtained from another by removing parameters), although extensions exist for non-nested models. Finally, AIC is a relative measure; it only compares models within a given set. It does not guarantee that the selected model is the "best" model in an absolute sense.
Closing Insights
The Akaike Information Criterion is a powerful and versatile tool for model selection across a wide range of statistical applications. Its ability to balance model fit and complexity makes it indispensable for building robust and generalizable models, leading to more accurate predictions and more reliable scientific inferences. Understanding AIC is not only crucial for researchers but also for anyone involved in data analysis, predictive modeling, and machine learning. While AIC has limitations, its widespread use and continuing development solidify its position as a cornerstone in statistical modeling. The future of AIC likely involves further refinement and integration with other model selection techniques to enhance its performance in diverse and complex data scenarios.
Exploring the Connection Between Model Interpretability and AIC
Model interpretability refers to the ease with which a model's predictions can be understood and explained. The connection between model interpretability and AIC is indirect but significant. While AIC doesn't directly assess interpretability, the model selection process guided by AIC often leads to models that are more interpretable. This is because AIC penalizes model complexity. Simpler models, favored by AIC in many cases, tend to be more interpretable than highly complex models with numerous interactions or non-linear terms. For example, in regression analysis, a simpler model with fewer predictors is generally easier to interpret than a complex model with many interactions. However, it's important to note that AIC doesn't guarantee interpretability; a model with low AIC might still be complex and difficult to interpret. Researchers often need to balance the benefits of a low AIC score with the need for a model that can be easily understood and explained in a given context.
Further Analysis of Model Interpretability
Model interpretability is a crucial aspect of model selection, particularly in applications where understanding the reasons behind predictions is essential. The importance of interpretability varies depending on the context. In some cases, prediction accuracy is paramount, even at the expense of interpretability (e.g., fraud detection). In other cases, understanding the underlying mechanisms is more crucial (e.g., medical diagnosis). The trade-off between prediction accuracy and interpretability is a key consideration in model selection, and there is no single "best" approach. Techniques like LASSO (Least Absolute Shrinkage and Selection Operator) regression can help promote both accurate predictions and interpretable models by shrinking less important coefficients towards zero, effectively simplifying the model.
Factor Affecting Interpretability | Description |
---|---|
Model Complexity | Simpler models (fewer parameters) are generally easier to interpret. |
Feature Engineering | Well-chosen features can lead to more interpretable models. |
Model Type | Some model types (e.g., linear regression) are inherently more interpretable than others (e.g., neural networks). |
Visualization Techniques | Effective visualizations can enhance the understanding of complex models. |
FAQ Section
-
Q: What is the difference between AIC and AICc? A: AIC is the standard Akaike Information Criterion, while AICc is a corrected version that accounts for bias in AIC, especially when the sample size is small relative to the number of parameters. AICc generally provides a more reliable estimate of the relative quality of models.
-
Q: Can I use AIC to compare models with different response variables? A: No, AIC can only be used to compare models that are fitted to the same dataset and have the same response variable.
-
Q: What should I do if two models have very similar AIC values? A: If the difference in AIC values is small (e.g., less than 2), the models are considered to be practically equivalent. Other factors, such as interpretability or computational cost, might guide the final choice.
-
Q: Is a lower AIC always better? A: Yes, within a set of models fitted to the same data, a lower AIC indicates a relatively better model in terms of balancing model fit and complexity.
-
Q: How does AIC handle non-nested models? A: While AIC is primarily designed for nested models, modifications and alternative approaches exist for comparing non-nested models. These approaches often involve comparing models based on their predictive performance on a hold-out dataset.
-
Q: What are some alternatives to AIC for model selection? A: Other model selection criteria include BIC (Bayesian Information Criterion), cross-validation, and adjusted R-squared (in the context of linear regression). The choice of criterion depends on the specific research question and the priorities of the analysis.
Practical Tips
-
Consider AICc for Small Samples: If your sample size is small relative to the number of parameters, always use AICc instead of AIC.
-
Compare Multiple Models: Don’t rely on a single model. Fit several candidate models and use AIC to compare them.
-
Visualize Results: Create plots or tables to clearly present the AIC values and compare models.
-
Use Appropriate Software: Statistical software packages (R, Python, SAS) provide functions for calculating AIC and AICc.
-
Don’t Overinterpret Small Differences: Small differences in AIC values may not be practically significant.
-
Consider Model Interpretability: While AIC focuses on predictive accuracy, also consider the interpretability of the chosen model.
-
Check Model Assumptions: Before relying on AIC, ensure that the assumptions of the chosen model are met.
-
Validate on Independent Data: After selecting a model using AIC, validate its performance on an independent dataset to assess its generalizability.
Final Conclusion
The Akaike Information Criterion stands as a cornerstone in the field of statistical model selection. Its ability to balance model fit with complexity, enabling the selection of models that are both accurate and parsimonious, has revolutionized the way researchers approach data analysis. While its application requires a thorough understanding of statistical principles, the benefits in terms of improved predictions, more reliable inferences, and a greater understanding of complex systems far outweigh the challenges. By mastering the principles of AIC and applying the practical tips discussed herein, researchers and data scientists can significantly enhance the rigor and effectiveness of their work, uncovering deeper insights from data and contributing to more impactful discoveries. Continued exploration of AIC and its refinements will undoubtedly further strengthen its role in navigating the complex landscape of statistical modeling.
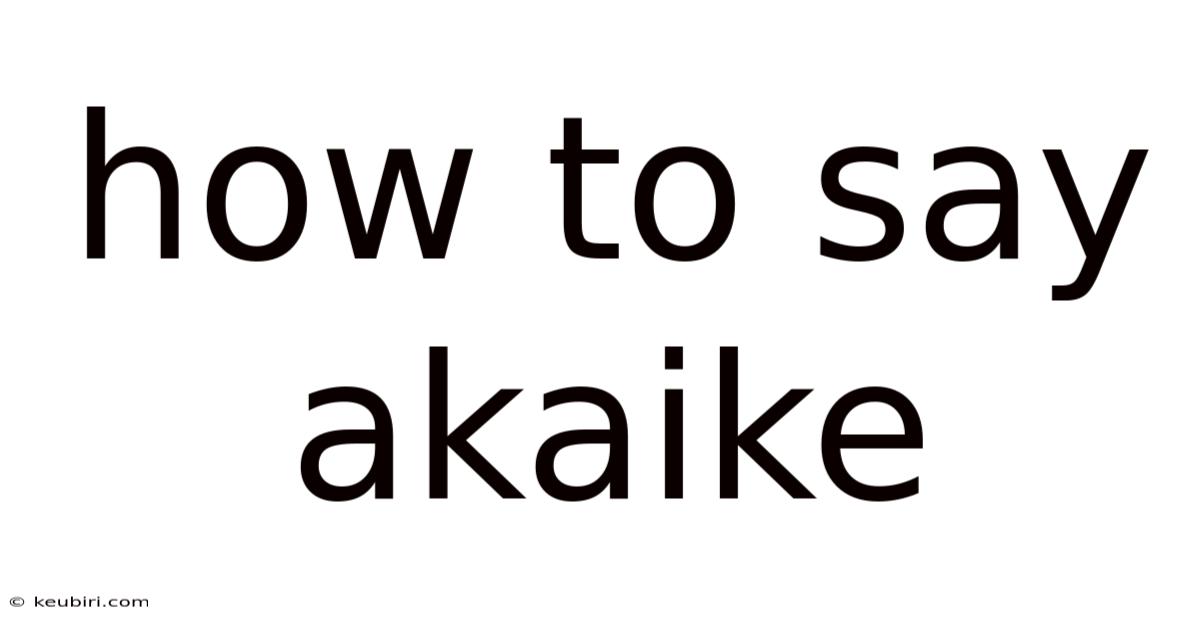
Thank you for visiting our website wich cover about How To Say Akaike. We hope the information provided has been useful to you. Feel free to contact us if you have any questions or need further assistance. See you next time and dont miss to bookmark.
Also read the following articles
Article Title | Date |
---|---|
How To Say Nipple In German | Apr 03, 2025 |
How To Say I Will Convey Your Message | Apr 03, 2025 |
How To Say To Your Best Friend I Love You | Apr 03, 2025 |
How To Say Swakopmund | Apr 03, 2025 |
How To Say Nunc Pro Tunc | Apr 03, 2025 |