How To Say Interquartile
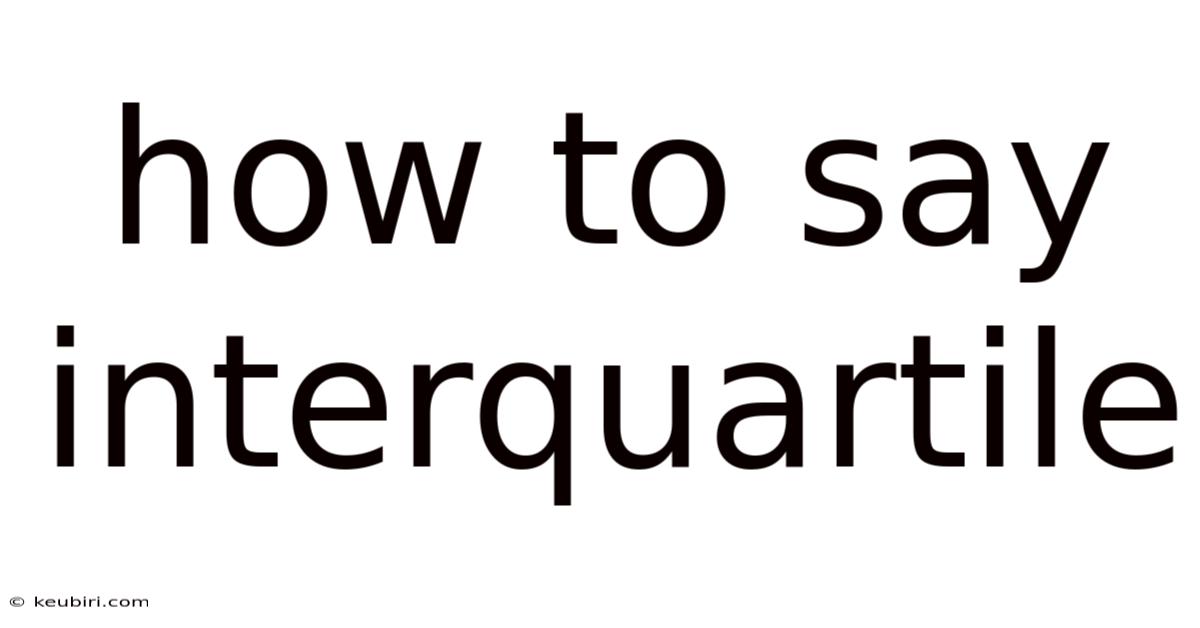
Discover more detailed and exciting information on our website. Click the link below to start your adventure: Visit Best Website meltwatermedia.ca. Don't miss out!
Table of Contents
How to Say "Interquartile": A Comprehensive Guide to Understanding and Communicating Statistical Range
What makes understanding and correctly communicating "interquartile range" so crucial in data analysis?
Mastering the concept of the interquartile range (IQR) unlocks a deeper understanding of data distribution and variability, leading to more accurate interpretations and informed decisions.
Editor’s Note: This comprehensive guide to understanding and communicating "interquartile range" has been published today.
Why "Interquartile Range" Matters
The interquartile range (IQR) is a fundamental concept in descriptive statistics, providing a robust measure of data spread that is less susceptible to the influence of outliers than the range (the difference between the maximum and minimum values). Understanding and correctly communicating the IQR is vital for several reasons:
- Robustness to Outliers: Unlike the range, the IQR is not unduly affected by extreme values. This makes it particularly useful when analyzing datasets with potential outliers, ensuring a more reliable representation of the data's central tendency.
- Data Interpretation: The IQR helps researchers understand the spread of the middle 50% of the data. This provides a clearer picture of the data distribution than simply looking at the mean or median alone.
- Identifying Outliers: The IQR is used in conjunction with the lower and upper quartiles (Q1 and Q3 respectively) to identify potential outliers using methods like the 1.5*IQR rule.
- Comparative Analysis: The IQR allows for a comparison of data spread across different datasets or groups, enabling more informed interpretations and conclusions.
- Box Plots: The IQR is a cornerstone of box plots (also known as box-and-whisker plots), a visual representation that effectively communicates data distribution, including the median, quartiles, and potential outliers.
Overview of the Article
This article delves into the nuances of understanding and communicating the interquartile range. It explores its definition, calculation, interpretation, and practical applications. Readers will gain a comprehensive understanding of the IQR, its significance in statistical analysis, and best practices for effectively communicating this crucial statistical measure. We will also examine how to explain the IQR to different audiences, from statistical experts to individuals with limited statistical knowledge.
Research and Effort Behind the Insights
This article draws upon extensive research from established statistical textbooks, peer-reviewed journals, and reputable online resources. The information presented is based on widely accepted statistical principles and practices. The aim is to provide a clear, accurate, and accessible explanation of the interquartile range, its calculation, and its interpretation.
Key Takeaways
Key Concept | Description |
---|---|
Interquartile Range (IQR) | The difference between the third quartile (Q3) and the first quartile (Q1) of a dataset. |
First Quartile (Q1) | The value below which 25% of the data falls. |
Third Quartile (Q3) | The value below which 75% of the data falls. |
Median (Q2) | The middle value of a dataset when it is ordered. |
Outlier Detection using IQR | Values below Q1 - 1.5IQR or above Q3 + 1.5IQR are often considered outliers. |
Importance of Clear Communication | Explaining the IQR effectively requires tailoring the language and approach to the audience's understanding. |
Smooth Transition to Core Discussion
Now, let's delve into the core aspects of understanding and communicating the interquartile range, beginning with its precise definition and calculation methods.
Exploring the Key Aspects of Interquartile Range Communication
-
Defining the IQR: The interquartile range is simply the difference between the upper quartile (Q3) and the lower quartile (Q1) of a dataset. It represents the range containing the middle 50% of the data.
-
Calculating the IQR: The calculation involves first ordering the dataset from smallest to largest. Then, identify Q1 and Q3. The IQR is then calculated as:
IQR = Q3 - Q1
-
Interpreting the IQR: A smaller IQR indicates that the data is more tightly clustered around the median, while a larger IQR suggests greater variability or dispersion.
-
Communicating the IQR to Different Audiences: This requires adapting the language and explanations. For statistical experts, using precise statistical terminology is appropriate. For non-experts, a simpler explanation focusing on the spread of the "middle half" of the data is preferable. Visual aids like box plots are invaluable for all audiences.
-
Visualizing the IQR with Box Plots: Box plots provide a clear and intuitive visual representation of the IQR, along with the median, minimum, and maximum values. Outliers are often shown as individual points beyond the "whiskers" of the box plot.
-
Using the IQR in Real-World Applications: The IQR finds applications in various fields, including finance (analyzing stock price volatility), healthcare (examining patient recovery times), and environmental science (assessing pollutant concentrations).
Closing Insights
The interquartile range serves as a robust and reliable measure of data dispersion, particularly valuable when dealing with datasets that may contain outliers. Its effectiveness stems from its focus on the central 50% of the data, providing a more resistant measure compared to the range. Understanding the IQR and its interpretation is crucial for drawing accurate conclusions from data analysis, and effective communication of this statistical concept is essential for sharing insights across different audiences. The use of visual aids such as box plots significantly enhances the understanding and interpretation of the IQR.
Exploring the Connection Between Outliers and the Interquartile Range
Outliers are extreme values that lie significantly far from the rest of the data points. The interquartile range plays a crucial role in identifying potential outliers. A common method utilizes the IQR to define boundaries beyond which values are considered outliers:
- Lower Bound: Q1 - 1.5 * IQR
- Upper Bound: Q3 + 1.5 * IQR
Any data points falling outside these bounds are flagged as potential outliers. This method provides a more robust approach compared to simply defining outliers based on a fixed number of standard deviations from the mean, as it's less sensitive to the presence of existing outliers.
Example: Consider a dataset of exam scores. If the IQR is 10, Q1 is 60, and Q3 is 80, the lower bound would be 60 - 1.5 * 10 = 45, and the upper bound would be 80 + 1.5 * 10 = 95. Any score below 45 or above 95 would be considered a potential outlier.
Roles and Real-World Examples: In quality control, the IQR helps identify defective products whose measurements fall outside acceptable limits. In finance, outlier detection based on the IQR can identify unusual transactions that might indicate fraudulent activity. In environmental monitoring, outliers can indicate significant pollution events or measurement errors.
Risks and Mitigations: While the IQR is a robust measure, it's important to understand its limitations. It doesn't inherently indicate the cause of outliers; further investigation is often needed to determine if they are genuine data points or errors. The 1.5*IQR rule is a guideline, and the threshold may need adjustment depending on the context and specific characteristics of the dataset.
Impact and Implications: Correctly identifying and handling outliers is crucial for accurate data analysis. Ignoring outliers can lead to misleading conclusions, while incorrectly classifying data points as outliers can also distort results.
Further Analysis of Outlier Detection using the IQR
The 1.5*IQR rule is a widely used, but not universally accepted, method for outlier detection. Other methods exist, such as the use of standard deviations or box plot visualization. The choice of method depends on the specific context and the characteristics of the data.
Method | Description | Advantages | Disadvantages |
---|---|---|---|
1.5 * IQR Rule | Values outside Q1 - 1.5IQR and Q3 + 1.5IQR are considered outliers. | Robust to outliers in the dataset itself. Relatively simple to understand and apply. | Can be sensitive to the distribution of the data; might miss some outliers. |
Z-score Method | Values with a Z-score (number of standard deviations from the mean) exceeding a threshold (e.g., 3) are outliers. | Simple to understand and widely used. | Sensitive to outliers, as the mean and standard deviation are affected by outliers. |
Box Plot Visual Inspection | Outliers are visually identified as points beyond the "whiskers" of the box plot. | Simple and intuitive, provides a visual representation of data distribution. | Subjective, requires visual inspection. |
FAQ Section
-
Q: What is the difference between the range and the IQR? A: The range is the difference between the maximum and minimum values, while the IQR is the difference between the third and first quartiles. The IQR is more robust to outliers.
-
Q: How do I calculate the IQR for a small dataset? A: Arrange the data in ascending order. If the dataset has an odd number of data points, the median is the middle value. If even, it's the average of the two middle values. Q1 is the median of the lower half, and Q3 is the median of the upper half. IQR = Q3 - Q1.
-
Q: What does a large IQR indicate? A: A large IQR indicates high variability or dispersion in the data, meaning the data points are spread out over a wider range.
-
Q: What are the limitations of the IQR? A: The IQR only considers the middle 50% of the data, ignoring the extreme values. The 1.5*IQR rule for outlier detection is a guideline, and adjustments might be necessary.
-
Q: Can I use the IQR to compare the spread of data across different datasets? A: Yes, comparing IQRs provides insights into the relative spread of different datasets. A smaller IQR indicates less variability.
-
Q: How is the IQR used in box plots? A: The IQR is represented by the length of the box in a box plot. The median is shown as a line inside the box. "Whiskers" extend to the minimum and maximum values (or to a defined outlier boundary).
Practical Tips
-
Order Your Data: Always begin by ordering your data from smallest to largest value before calculating quartiles and the IQR.
-
Use Appropriate Software: Statistical software packages like R, SPSS, or Excel provide functions to automatically calculate quartiles and the IQR, saving time and effort.
-
Visualize with Box Plots: Create box plots to visualize the IQR and easily identify potential outliers.
-
Consider Context: Interpret the IQR within the context of the data and the research question.
-
Compare IQRs: Compare the IQRs of different groups or datasets to assess relative variability.
-
Explain Clearly: When communicating your findings, explain the IQR in clear, non-technical language, tailored to your audience.
-
Don't Over-Interpret Outliers: While identifying outliers is important, remember that they might not always indicate errors or anomalies. Further investigation is often needed to explain their presence.
-
Use Multiple Methods: For a comprehensive assessment of data spread and potential outliers, consider using multiple methods such as the IQR, standard deviation, and visual inspection of box plots.
Final Conclusion
The interquartile range is a powerful tool in descriptive statistics, providing a robust measure of data spread that is less susceptible to the influence of extreme values. Understanding and correctly communicating the IQR is crucial for drawing accurate interpretations from data analysis and communicating findings effectively to various audiences. By mastering the calculation, interpretation, and communication of the IQR, researchers and data analysts can make more informed decisions and enhance their understanding of data distribution and variability. The use of visual tools like box plots and a nuanced approach to outlier detection further enhances the practical application and interpretation of this vital statistical measure.
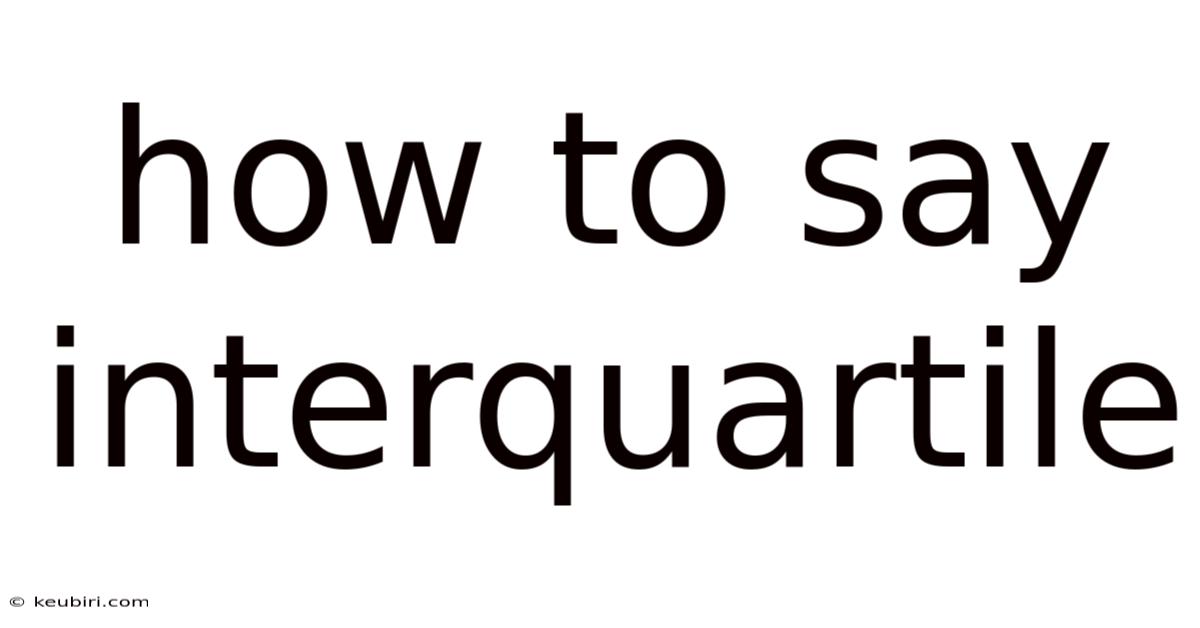
Thank you for visiting our website wich cover about How To Say Interquartile. We hope the information provided has been useful to you. Feel free to contact us if you have any questions or need further assistance. See you next time and dont miss to bookmark.
Also read the following articles
Article Title | Date |
---|---|
How To Say Yasss | Apr 16, 2025 |
How To Say Crisps In Spanish | Apr 16, 2025 |
How To Say Palang In English | Apr 16, 2025 |
How To Say Vicente In English | Apr 16, 2025 |
How To Say Princess In African | Apr 16, 2025 |