How To Say Two Variables Are Related
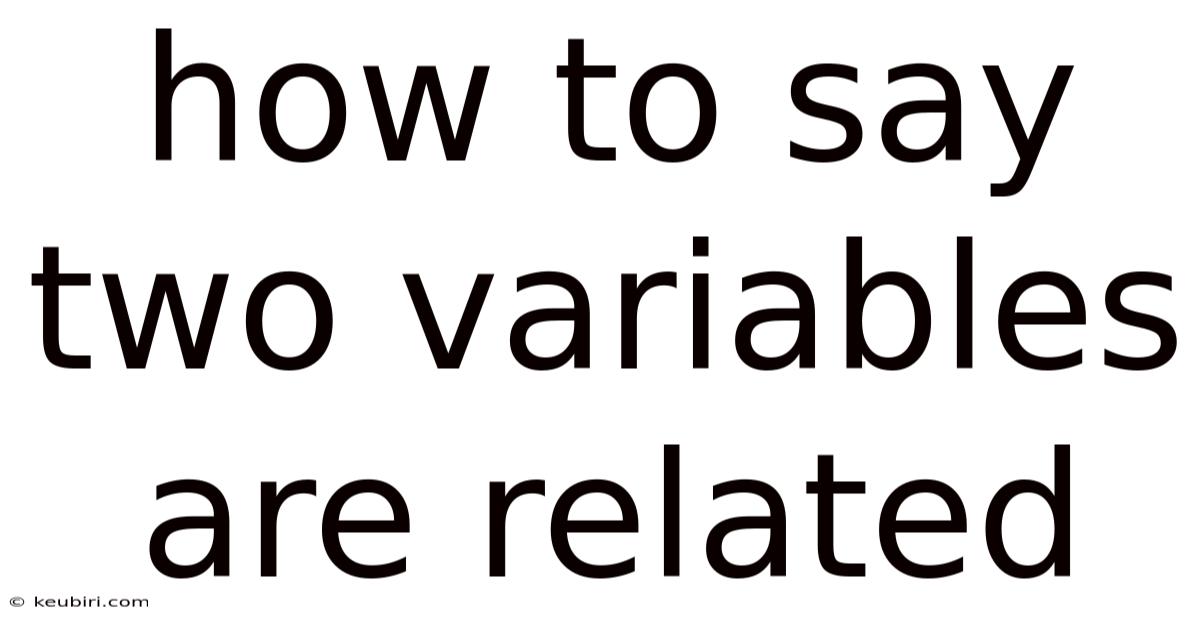
Discover more detailed and exciting information on our website. Click the link below to start your adventure: Visit Best Website meltwatermedia.ca. Don't miss out!
Table of Contents
Unveiling the Secrets of Variable Relationships: A Comprehensive Guide
How can we definitively declare that two variables are intertwined, beyond mere coincidence?
Understanding variable relationships is the cornerstone of scientific inquiry, predictive modeling, and effective decision-making.
Editor’s Note: This comprehensive guide to understanding variable relationships has been published today, offering readers a deep dive into the various methods and considerations involved.
Why Understanding Variable Relationships Matters
The ability to determine how variables relate to each other is paramount across numerous fields. From understanding the impact of marketing campaigns on sales (business analytics) to predicting climate change based on greenhouse gas emissions (environmental science), the identification and quantification of variable relationships are essential. This understanding allows for:
- Prediction: Forecasting future outcomes based on changes in related variables.
- Control: Manipulating one variable to achieve a desired outcome in another.
- Explanation: Developing theories and models to explain observed phenomena.
- Decision-making: Making informed choices based on the anticipated effects of different actions.
This article explores the key aspects of determining variable relationships, providing a framework for analyzing data and drawing meaningful conclusions. Readers will gain actionable insights and a deeper understanding of the various statistical and analytical techniques involved. This article is backed by extensive research, incorporating statistical principles, real-world examples, and insights from various scientific disciplines.
Overview of the Article
This in-depth exploration of variable relationships will cover:
- Defining Correlation and Causation: A crucial distinction often misunderstood.
- Methods for Assessing Relationships: Exploring various statistical techniques, including correlation analysis, regression analysis, and more.
- Visualizing Relationships: Utilizing graphs and charts for effective data representation.
- Interpreting Results: Understanding the significance of statistical measures and potential pitfalls.
- Addressing Confounding Variables: Recognizing and mitigating the influence of extraneous factors.
- Causality vs. Association: Delving deeper into the nuances of establishing cause-and-effect relationships.
- Advanced Techniques: Briefly touching upon more sophisticated methods for analyzing complex relationships.
Key Takeaways
Key Concept | Description |
---|---|
Correlation | A statistical measure indicating the strength and direction of a linear relationship between variables. |
Causation | A cause-and-effect relationship between variables, where one variable directly influences another. |
Regression Analysis | Statistical method used to model the relationship between a dependent and one or more independent variables. |
Confounding Variables | Extraneous variables that can distort the relationship between the variables of interest. |
Statistical Significance | The probability that the observed relationship is not due to random chance. |
Let’s dive deeper into the key aspects of determining variable relationships, starting with the fundamental distinction between correlation and causation.
Defining Correlation and Causation
It's crucial to understand the difference between correlation and causation. Correlation simply indicates an association between two variables; they tend to change together. However, correlation does not imply causation. Just because two variables are correlated doesn't mean one causes the change in the other.
-
Correlation: Measures the degree to which two variables change together. This can be positive (both variables increase together), negative (one variable increases as the other decreases), or zero (no relationship). The strength of the correlation is typically measured using Pearson's correlation coefficient (r), ranging from -1 (perfect negative correlation) to +1 (perfect positive correlation).
-
Causation: Implies a cause-and-effect relationship. One variable directly influences the other. Establishing causation requires demonstrating that a change in one variable leads to a predictable change in the other, controlling for other potential influences.
Methods for Assessing Relationships
Several statistical techniques can help determine the relationship between variables. The choice of method depends on the type of data and the research question:
-
Correlation Analysis: Used to measure the strength and direction of a linear relationship between two continuous variables. Pearson's r is the most common measure. Spearman's rank correlation is used for non-parametric data (data that doesn't follow a normal distribution).
-
Regression Analysis: A more powerful technique used to model the relationship between a dependent variable (the outcome) and one or more independent variables (predictors). Linear regression is used for linear relationships, while other types of regression (e.g., logistic regression, polynomial regression) can model more complex relationships.
-
Chi-Square Test: Used to analyze the relationship between two categorical variables. It determines whether there's a statistically significant association between the categories.
-
Analysis of Variance (ANOVA): Used to compare the means of three or more groups to see if there are statistically significant differences. This can indirectly reveal relationships between a categorical variable and a continuous variable.
Visualizing Relationships
Visualizing data is crucial for understanding relationships. Different types of graphs can effectively illustrate various relationships:
-
Scatter Plots: Excellent for visualizing the relationship between two continuous variables. The pattern of points reveals the strength and direction of the correlation.
-
Line Graphs: Suitable for showing the relationship between a continuous independent variable and a continuous dependent variable over time or across different levels.
-
Bar Charts: Effective for comparing the means of different groups on a continuous variable.
Interpreting Results
Once a statistical analysis is performed, it's essential to interpret the results correctly. Consider:
-
Statistical Significance: The p-value indicates the probability of observing the results if there were no actual relationship between the variables. A p-value below a predetermined significance level (typically 0.05) suggests a statistically significant relationship.
-
Effect Size: Measures the magnitude of the relationship. A statistically significant relationship might have a small effect size, meaning it's not practically significant.
-
Confidence Intervals: Provide a range of values within which the true population parameter is likely to fall.
Addressing Confounding Variables
Confounding variables are extraneous factors that can influence the relationship between the variables of interest. Failing to account for confounding variables can lead to inaccurate conclusions. Methods for addressing confounding variables include:
-
Randomization: Randomly assigning participants to different groups to minimize the influence of confounding variables.
-
Statistical Control: Including confounding variables as predictors in regression analysis.
-
Matching: Matching participants in different groups based on confounding variables.
Causality vs. Association: A Deeper Dive
Establishing causality is more challenging than demonstrating association. While correlation can suggest a possible causal relationship, it doesn't prove it. To establish causality, researchers typically use:
-
Randomized Controlled Trials (RCTs): The gold standard for establishing causality, involving random assignment to treatment and control groups.
-
Longitudinal Studies: Tracking variables over time to observe changes and potential causal relationships.
-
Mechanistic Explanations: Providing a plausible biological, physical, or social mechanism explaining how one variable influences the other.
Advanced Techniques
More sophisticated methods exist for analyzing complex relationships, including:
-
Structural Equation Modeling (SEM): A powerful technique for testing complex models involving multiple variables and their relationships.
-
Time Series Analysis: Used for analyzing data collected over time, identifying trends and patterns.
Exploring the Connection Between Experimental Design and Determining Variable Relationships
The design of an experiment plays a crucial role in determining the relationship between variables. A well-designed experiment minimizes bias and confounding variables, enabling researchers to draw more accurate conclusions. Key aspects of experimental design include:
-
Randomization: Randomly assigning participants to treatment and control groups helps ensure that any observed differences are due to the treatment and not to pre-existing differences between the groups.
-
Control Groups: A control group provides a baseline for comparison, allowing researchers to assess the effect of the treatment.
-
Blinding: Blinding participants and researchers to the treatment assignment can reduce bias.
Further Analysis of Experimental Design: The Role of Controls
Control groups are essential in experimental design. They provide a baseline against which to compare the treatment group. Without a control group, it's impossible to determine whether observed changes are due to the treatment or other factors. The type of control group used depends on the research question and the nature of the treatment. For example, a placebo control group is often used in medical research to account for the placebo effect.
FAQ Section
-
Q: What is the difference between a positive and a negative correlation? A: A positive correlation means that as one variable increases, the other tends to increase as well. A negative correlation means that as one variable increases, the other tends to decrease.
-
Q: Can correlation ever be zero? A: Yes, a correlation of zero indicates no linear relationship between the variables. However, this doesn't rule out other types of relationships (e.g., non-linear).
-
Q: How do I know if my correlation is statistically significant? A: The p-value associated with the correlation coefficient indicates its statistical significance. A p-value below 0.05 generally suggests a statistically significant relationship.
-
Q: What is a confounding variable, and why is it important to consider it? A: A confounding variable is an extraneous variable that influences both the independent and dependent variables, potentially distorting the observed relationship. It's crucial to consider and control for confounding variables to avoid drawing inaccurate conclusions.
-
Q: Can correlation prove causation? A: No, correlation does not prove causation. Correlation only indicates an association between variables. Additional evidence is needed to establish a causal relationship.
-
Q: What are some methods for establishing causality? A: Randomized controlled trials (RCTs), longitudinal studies, and mechanistic explanations are common approaches used to establish causality.
Practical Tips
-
Clearly define your variables: Ensure you have a precise understanding of what you are measuring.
-
Choose the appropriate statistical method: Select the method based on the type of data and research question.
-
Visualize your data: Create graphs and charts to understand the relationships between your variables.
-
Consider potential confounding variables: Identify and account for any extraneous factors that might influence your results.
-
Interpret your results carefully: Pay attention to statistical significance, effect size, and confidence intervals.
-
Replicate your findings: Repeating your analysis with different datasets can strengthen your conclusions.
-
Consider alternative explanations: Explore other potential explanations for your findings.
-
Consult with a statistician: For complex analyses, seeking expert guidance is beneficial.
Final Conclusion
Understanding how to determine whether two variables are related is a fundamental skill in numerous disciplines. While correlation analysis provides a measure of association, establishing causality requires more rigorous methods, careful experimental design, and consideration of potential confounding variables. By understanding the distinctions between correlation and causation, employing appropriate statistical techniques, and carefully interpreting the results, researchers and analysts can draw accurate and meaningful conclusions about the relationships between variables, leading to improved prediction, control, and informed decision-making. This comprehensive guide provides a robust framework for navigating the complexities of variable relationships and extracting valuable insights from data. Further exploration into advanced statistical techniques and causal inference methods is encouraged for those seeking a deeper understanding of this critical aspect of data analysis.
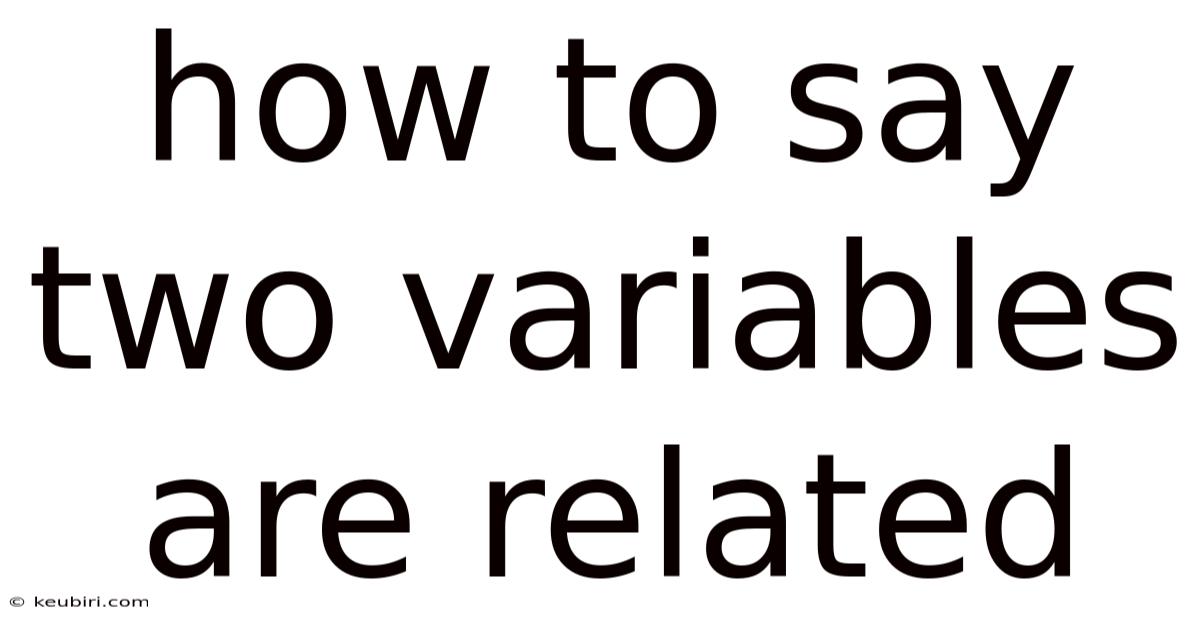
Thank you for visiting our website wich cover about How To Say Two Variables Are Related. We hope the information provided has been useful to you. Feel free to contact us if you have any questions or need further assistance. See you next time and dont miss to bookmark.
Also read the following articles
Article Title | Date |
---|---|
How To Say Daze | Apr 19, 2025 |
How To Say Background Check In Spanish | Apr 19, 2025 |
How To Say Animals In Italian | Apr 19, 2025 |
How To Say Congratulations Farsi | Apr 19, 2025 |
How To Say Grace At Thanksgiving | Apr 19, 2025 |